Hi, I'm Yan Cui
I'm an AWS Serverless Hero. I provide training and consulting on AWS and serverless application.
I have run production workloads at scale in AWS since 2010 and have a track record of improving businesses with technology.
I have been an architect and principal engineer within a variety of industries including banking, e-commerce, social networks, live streaming and mobile gaming.
I have worked on large scale systems that handle millions of concurrent users and process billions of events per day.
Nowadays I help client launch their product ideas, improve their development processes and upskill their teams.
Want to work together? Get in touch.
" Yan's time was easily the best investment I've made in tech. He instantly understood our complex requirements and provided creative and practical solutions that we were able to implement the same day. Yan's expertise in AWS is world-class. Very highly recommended. "
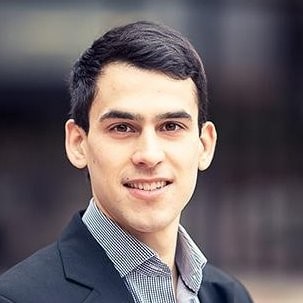
Iman Fadaei
Founder at Whitelance
" Yan was a key factor for the success of the launch of our app. He cared about every detail and took his time to understand each task. Thanks to him, we made better decisions that made us stay on the budget, keep the app safe and go even faster. "
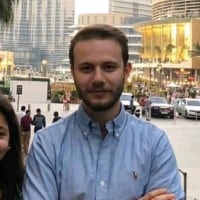
Karam Fattal
Co-Founder at Nova Sport
" If you want to learn Serverless and AWS, you can not find a better person than Yan. His workshop is full of practical, actionable advice. This is one of the best investments you can make for your Serverless journey. "
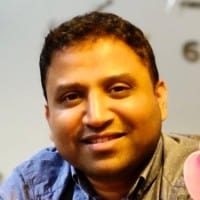
Bala Chalasani
Founder at Cloud Architectures
Let’s connect
I’m posting AWS and serverless contents on multiple Social Media platforms. Reach out and let’s connect.
Become a better AWS Developer
Join 8,000+ engineers who are improving their AWS and serverless skills every Monday.