
Yan Cui
I help clients go faster for less using serverless technologies.
AWS offers a wealth of options for implementing messaging patterns such as pub-sub with Lambda, let’s compare and contrast some of these options.
The pub-sub pattern
Publish-Subscribe
(often shortened to pub-sub) is a messaging pattern where publishers and subscribers are decoupled through an intermediary broker (ZeroMQ, RabbitMQ, SNS, etc.).

SNS + Lambda
In the AWS ecosystem, the obvious candidate for the broker role is SNS.
SNS will make 3 attempts for your function to process a message before sending it to a Dead Letter Queue (DLQ) if a DLQ is specified for the function. However, according to an analysis by the folks at OpsGenie, the no. of retries can be as many as 6.
Another thing to consider is the degree of parallelism this setup offers. For each message SNS will create a new invocation of your function. So if you publish 100 messages to SNS then you can have 100 concurrent executions of the subscribed Lambda function.
This is great if you’re optimising for throughput.
However, we’re often constrained by the max throughput our downstream dependencies can handle – databases, S3, internal/external services, etc.
If the burst in throughput is short then there’s a good chance the retries would be sufficient (there’s a randomised, exponential back off between retries too) and you won’t miss any messages.
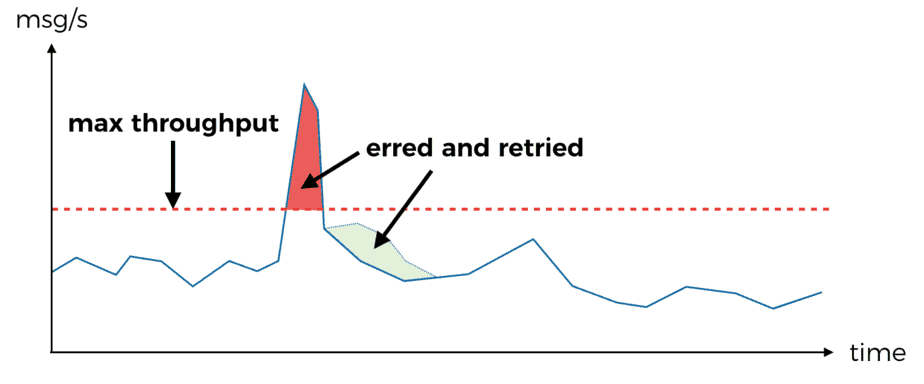
If the burst in throughput is sustained over a long period of time, then you can exhaust the max no. of retries. At this point you’ll have to rely on the DLQ and possibly human intervention in order to recover the messages that couldn’t be processed the first time round.
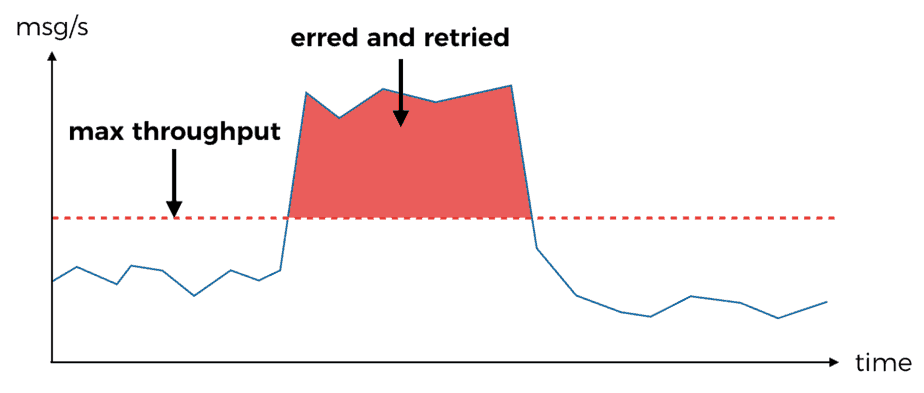
Similarly, if the downstream dependency experiences an outage then all messages received and retried during the outage are bound to fail.
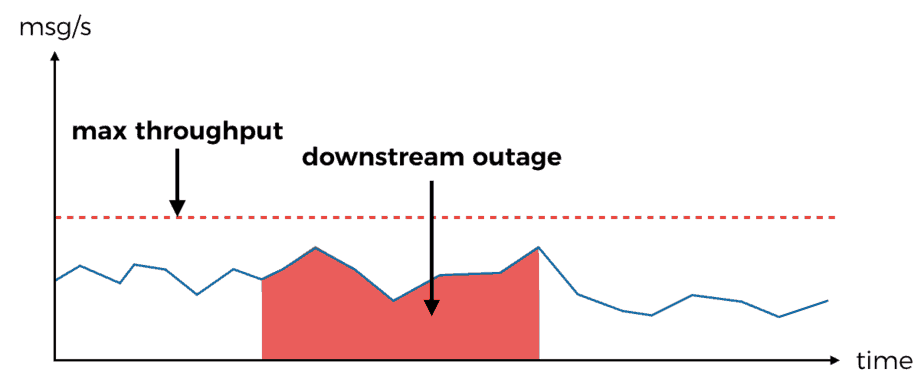
You can also run into Lambda limit on no. of concurrent executions in a region. Since this is an account wide limit, it will also impact your other systems that rely on AWS Lambda – APIs, event processing, cron jobs, etc.
Kinesis Streams + Lambda
Kinesis Streams differ from SNS in many ways:
- Lambda polls Kinesis for records up to 5 times a second, whereas SNS would push messages to Lambda
- records are received in batches (up to your specified maximum), SNS invokes your function with one message
- if your function returns an error or times out, then you’ll keep receiving the same batch of records until you either successfully process them or the data are no longer available in the stream
- the degree of parallelism is determined by the no. of shards in the stream as there is one dedicated invocation per shard
- Kinesis Streams are charged based on no. of records pushed to the stream; shard hours, and whether or not you enable extended retention
SNS is prone to suffer from temporal issues – bursts in traffic, downstream outage, etc. Kinesis on the other hand deals with these issues much better.
- degree of parallelism is constrained by no. of shards, which can be used to amortise bursts in message rate
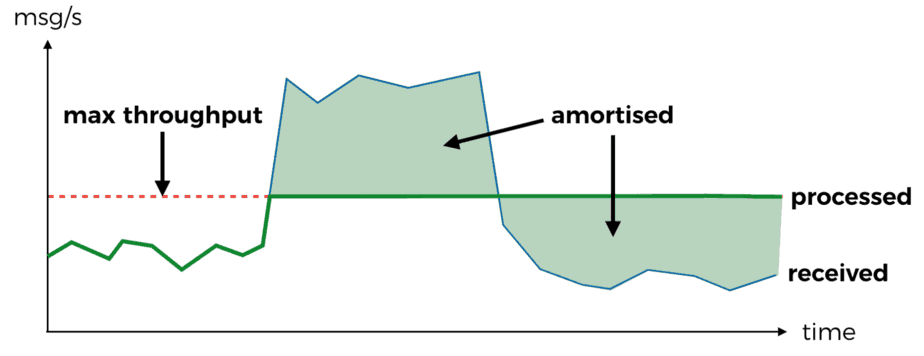
- records are retried until success, unless the outage lasts longer than the retention policy you have on the stream (default is 24 hours) you will eventually be able to process the records
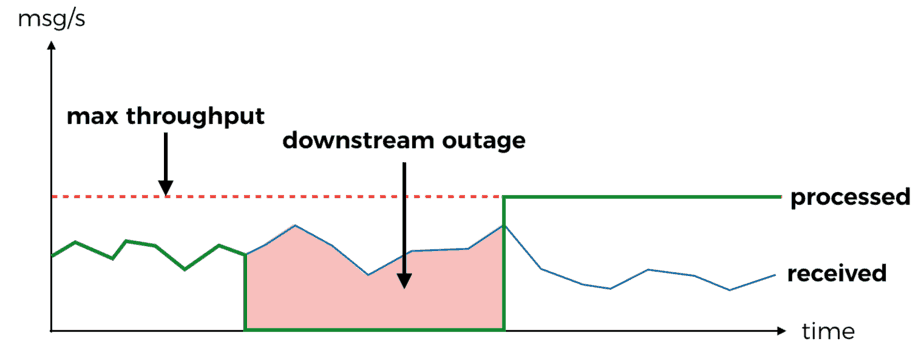
But Kinesis Streams is not without its own problems. In fact, from my experience using Kinesis Streams with Lambda I have found a no. of caveats that we needed to understand in order to make effective use of them.
You can read about these caveats here.
There are also several operational considerations to take into account:
- because Kinesis Streams is charged (in part) based on shard hours, so a dormant stream would have a baseline cost of $0.015 per shard per hour (~$11 per shard per month)
- there is no built-in auto-scaling capability for Kinesis Streams neither, so there is also additional management overhead for scaling them up based on utilization
It is possible to build auto-scaling capability yourself, which I had done at my previous (failed) startup. Whilst I can’t share the code you can read about the approach and my design thinking here.
Interestingly, Kinesis Streams is not the only streaming option available on AWS, there is also DynamoDB Streams.
DynamoDB Streams + Lambda
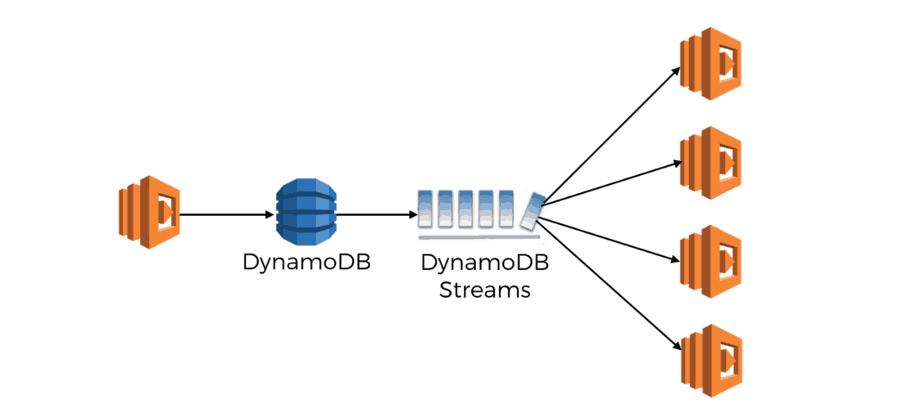
By and large, DynamoDB Streams + Lambda works the same way as Kinesis Streams + Lambda. Operationally, it does have some interesting twists:
- DynamoDB Streams auto-scales the no. of shards
- if you’re processing DynamoDB Streams with AWS Lambda then you don’t pay for the reads from DynamoDB Streams (but you still pay for the read & write capacity units for the DynamoDB table itself)
- Kinesis Streams offers the option to extend data retention to 7 days; DynamoDB Streams doesn’t offer such option
The fact that DynamoDB Streams auto-scales the no. of shards can be a double-edged sword. On one hand it eliminates the need for you to manage and scale the stream (or come up with home baked auto-scaling solution); on the other hand, it can also diminish the ability to amortize spikes in load you pass on to downstream systems.
AFAIK there is no way to limit the no. of shards a DynamoDB stream can scale up to – something you’d surely consider when implementing your own auto-scaling solution.
Should I use Kinesis or DynamoDB Streams?
I think the most pertinent question is “what is your source of truth?”
Does a row being written in DynamoDB make it canon to the state of your system? This is certainly the case in most N-tier systems that are built around a database, regardless whether it’s RDBMS or NoSQL.
In an event sourced system where state is modelled as a sequence of events (as opposed to a snapshot) the source of truth might well be the Kinesis stream – as soon as an event is written to the stream it’s considered canon to the state of the system.
Then, there’re other considerations around cost, auto-scaling, etc.
From a development point of view, DynamoDB Streams also has some limitations & shortcoming:
- each stream is limited to events from one table
- the records describe DynamoDB events and not events from your domain, which I always felt creates a sense of dissonance when I’m working with these events
Cost Implication of your Broker choice
Excluding the cost of Lambda invocations for processing the messages, here are some cost projections for using SNS vs Kinesis Streams vs DynamoDB Streams as the broker. I’m making the assumption that throughput is consistent, and that each message is 1KB in size.
monthly cost at 1 msg/s
monthly cost at 1,000 msg/s
These projections should not be taken at face value. For starters, the assumptions about a perfectly consistent throughput and message size is unrealistic, and you’ll need some headroom with Kinesis & DynamoDB Streams even if you’re not hitting the throttling limits.
That said, what these projections do tell me is that:
- you get an awful lot with each shard in Kinesis Streams
- whilst there’s a baseline cost for using Kinesis Streams, the cost grows much slower with scale compared to SNS and DynamoDB Streams, thanks to the significantly lower cost per million requests
Stacking it up
Whilst SNS, Kinesis & DynamoDB Streams are your basic choices for the broker, the Lambda functions can also act as brokers in their own right and propagate events to other services.
This is the approach used by the aws-lambda-fanout project from awslabs. It allows you to propagate events from Kinesis and DynamoDB Streams to other services that cannot directly subscribe to the 3 basic choice of brokers either because account/region limitations, or that they’re just not supported.
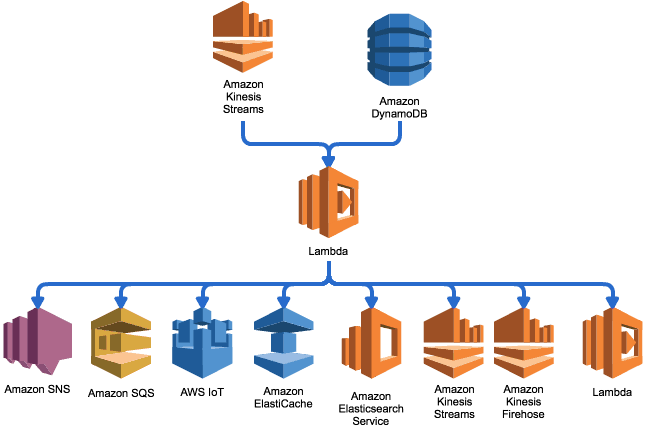
Whilst it’s a nice idea and definitely meets some specific needs, it’s worth bearing in mind the extra complexities it introduces – handling partial failures, dealing with downstream outages, misconfigurations, etc.
Conclusions
So what is the best event source for doing pub-sub with AWS Lambda? Like most tech decisions, it depends on the problem you’re trying to solve, and the constraints you’re working with.
In this post, we looked at SNS, Kinesis Streams and DynamoDB Streams as candidates for the broker role. We walked through a number of scenarios to see how the choice of event source affects scalability, parallelism, resilience against temporal issues and cost.
You should now have a much better understanding of the tradeoffs between these event sources when working with Lambda. In the next post, we will look at another popular messaging pattern, push-pull, and how we can implement it using Lambda. Again, we will look at a number of different services you can use and compare them.
Until next time!
Whenever you’re ready, here are 3 ways I can help you:
- Production-Ready Serverless: Join 20+ AWS Heroes & Community Builders and 1000+ other students in levelling up your serverless game. This is your one-stop shop to level up your serverless skills quickly.
- Do you want to know how to test serverless architectures with a fast dev & test loop? Check out my latest course, Testing Serverless Architectures and learn the smart way to test serverless.
- I help clients launch product ideas, improve their development processes and upskill their teams. If you’d like to work together, then let’s get in touch.