
Yan Cui
I help clients go faster for less using serverless technologies.
This article is brought to you by
The real-time data platform that empowers developers to build innovative products faster and more reliably than ever before.
This is the first of a multipart series that explores ideas on how we could apply the principles of chaos engineering to serverless architectures built around Lambda functions.
- part 1: how can we apply principles of chaos engineering to Lambda? <- you’re here
- part 2: latency injection for APIs
- part 3: fault injection for Lambda functions
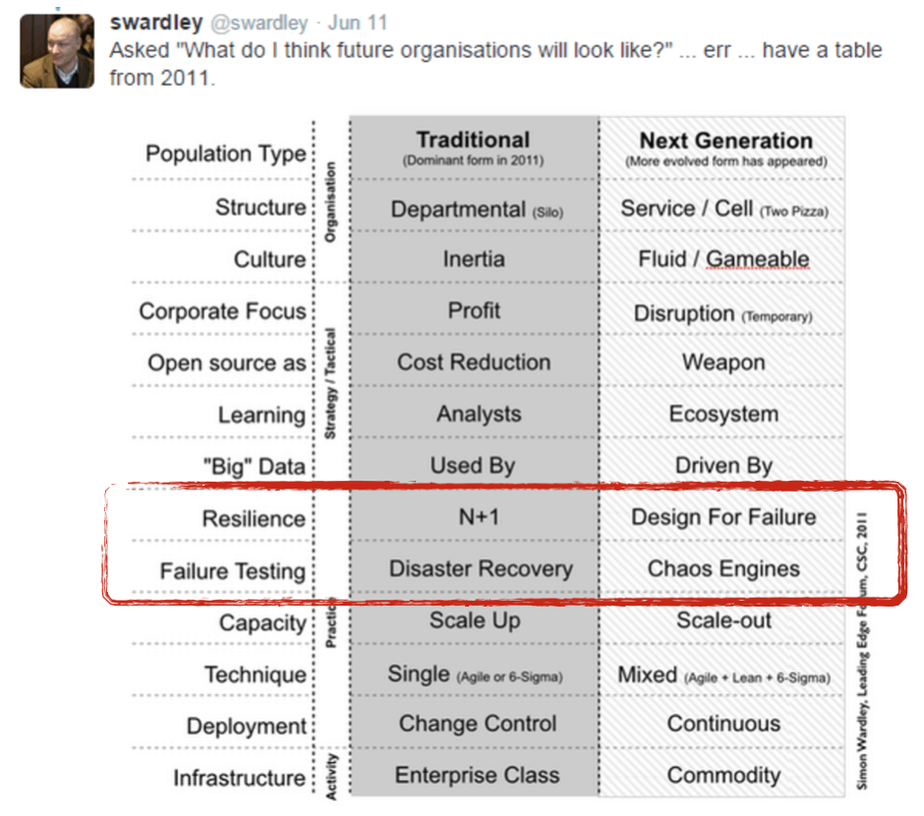
There’s no question about it, Netflix has popularised the principles of chaos engineering. By open sourcing some of their tools – notably the Simian Army – they have also helped others build confidence in their system’s capability to withstand turbulent conditions in production.
There seems to be a renewed interest in chaos engineering recently. As Russ Miles noted in a recent post, perhaps many companies have finally come to understand that chaos engineering is not about “hurting production”, but to build better understanding of, and confidence in a system’s resilience through controlled experiments.
This trend has been helped by the valuable (and freely available) information that Netflix has published, such as the Chaos Engineering e-book, and principlesofchaos.org.
Tools such as chaos-lambda by Shoreditch Ops (the folks behind the Artillery load test tool) look to replicate Netflix’s Chaos Monkey, but execute from inside a Lambda function instead of an EC2 instance – ence bringing you the cost saving and convenience Lambda offers.
I want to ask a different question however: how can one apply the principles of chaos engineering and some of the current practices to a serverless architecture comprised of Lambda functions?
When your system runs on EC2 instances, then naturally, you build resilience by designing for the most likely failure mode – server crashes (due to both hardware and software issues). Hence, a controlled experiment to validate the resilience of your system would artificially recreate the failure scenario by terminating EC2 instances, and then AZs, and then entire regions.
AWS Lambda, however, is a higher-level abstraction and has different failure modes to its EC2 counterparts. Hypothesis that focus on “what if we lose these EC2 instances” no longer apply as the platform handles these failure modes for you out of the box.
We need to ask different questions in order to understand the weaknesses within our serverless architectures.
More inherent chaos, not less
“We need to identify weaknesses before they manifest in system-wide, aberrant behaviors. Systemic weaknesses could take the form of: improper fallback settings when a service is unavailable; retry storms from improperly tuned timeouts; outages when a downstream dependency receives too much traffic; cascading failures when a single point of failure crashes; etc. We must address the most significant weaknesses proactively, before they affect our customers in production. We need a way to manage the chaos inherent in these systems, take advantage of increasing flexibility and velocity, and have confidence in our production deployments despite the complexity that they represent.”
Having built and operated a non-trivial serverless architecture, I have some understanding of the dangers awaiting you in this new world.
If anything, there are a lot more inherent chaos and complexity in these systems built around Lambda functions.
- modularity (unit of deployment) shifts from “services” to “functions”, and there are a lot more of them
- it’s harder to harden around the boundaries, because you need to harden around each function as opposed to a service which encapsulates a set of related functionalities
- there are more intermediary services (eg. Kinesis, SNS, API Gateway just to name a few), each with their own failure modes
- there are more configurations overall (timeout, IAM permissions, etc.), and therefore more opportunities for misconfiguration
Also, since we have traded off more control of our infrastructure* it means we now face more unknown failure modes** and often there’s little we can do when an outage does occur***.
* For better scalability, availability, cost efficiency and more convenience, which I for one, think it’s a fair trade in most cases.
** Everything the platform does for you – scheduling containers, scaling, polling Kinesis, retry failed invocations, etc. – have their own failure modes. These are often not obvious to us since they’re implementation details that are typically undocumented and are prone to change without notice.
*** For example, if an outage happens and prevents Lambda functions from processing Kinesis events, then we have no meaningful alternative than to wait for AWS to fix the problem. Since the current position on the shards is abstracted away and unavailable to us, we can’t even replace the Lambda functions with KCL processors that run on EC2.
Applying chaos to AWS Lambda
A good exercise regime would continuously push you to your limits but never actually put you over the limit and cause injury. If there’s an exercise that is clearly beyond your current abilities then surely you would not attempt it as the only possible outcome is getting yourself hurt!
The same common sense should be applied when designing controlled experiments for your serverless architecture. In order to “know” what the experiments tell us about the resilience of our system we also need to decide what metrics to monitor – ideally using client-side metrics, since the most important metric is the quality of service our users experience.
There are plenty of failure modes that we know about and can design for, and we can run simple experiments to validate our design. For example, since a serverless architecture is (almost always) also a microservice architecture, many of its inherent failure modes still apply:
- improperly tuned timeouts, especially for intermediate services, which can cause services at the edge to also timeout
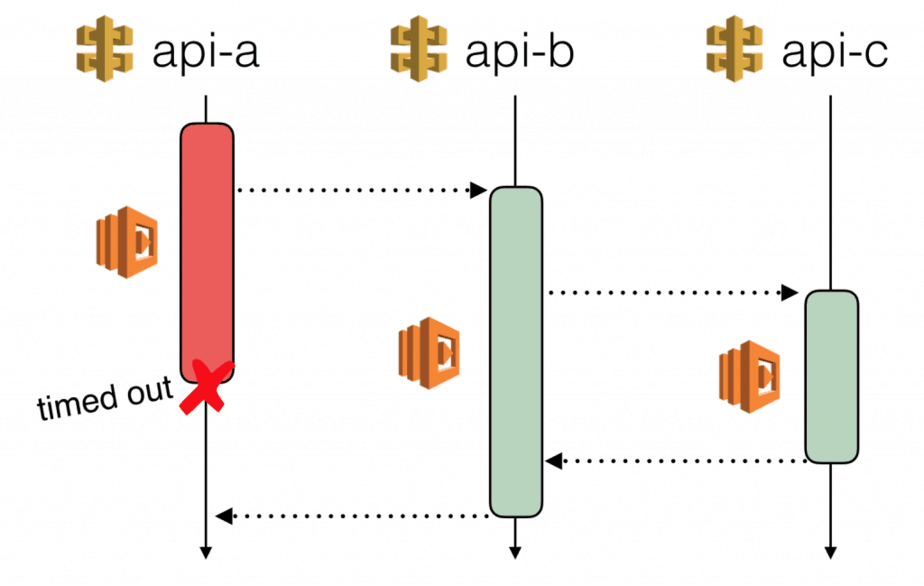
- missing error handling, which allows exceptions from downstream services to escape
- missing fallbacks for when a downstream service is unavailable or experiences an outage
Over the next couple of posts, we will explore how we can apply the practices of latency and fault injection to Lambda functions in order to simulate these failure modes and validate our design.
Further readings:
Whenever you’re ready, here are 4 ways I can help you:
- Production-Ready Serverless: Join 20+ AWS Heroes & Community Builders and 1000+ other students in levelling up your serverless game. This is your one-stop shop for quickly levelling up your serverless skills.
- Do you want to know how to test serverless architectures with a fast dev & test loop? Check out my latest course, Testing Serverless Architectures and learn the smart way to test serverless.
- I help clients launch product ideas, improve their development processes and upskill their teams. If you’d like to work together, then let’s get in touch.
- Join my community on Discord, ask questions, and join the discussion on all things AWS and Serverless.