
Yan Cui
I help clients go faster for less using serverless technologies.
This article is brought to you by
The real-time data platform that empowers developers to build innovative products faster and more reliably than ever before.
In the last post, we look at how you can implement pub-sub with AWS Lambda. We compared several event sources you can use, SNS, Kinesis streams and DynamoDB streams, and the tradeoffs available to you.
Let’s look at another messaging pattern today, push-pull, which is often referred to as fan-out/fan-in.
It’s really two separate patterns working in tandem.
Fan-out is often used on its own, where messages are delivered to a pool of workers in a round-robin fashion and each message is delivered to only one worker.
This is useful in at least two different ways:
- having a pool of workers to carry out the actual work allows for parallel processing and lead to increased throughput
- if each message represents an expensive task that can be broken down into smaller subtasks that can be carried out in parallel
In the second case where the original task (say, a batch job) is partitioned into many subtasks, you’ll need fan-in to collect result from individual workers and aggregate them together.
fan-out with SNS
As discussed above, SNS’s invocation per message policy is a good fit here as we’re optimizing for throughput and parallelism during the fan-out stage.
Here, a ventilator
function would partition the expensive task into subtasks, and publish a message to the SNS topic for each subtask.
This is essentially the approach we took when we implemented the timeline feature at Yubl (the last startup I worked at) which works the same as Twitter’s timeline – when you publish a new post it is distributed to your followers’ timeline; and when you follow another user, their posts would show up in your timeline shortly after.
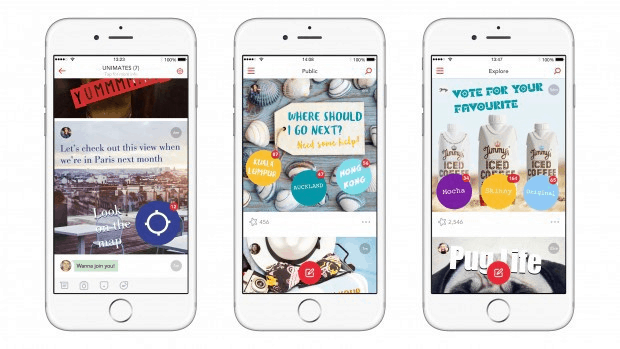
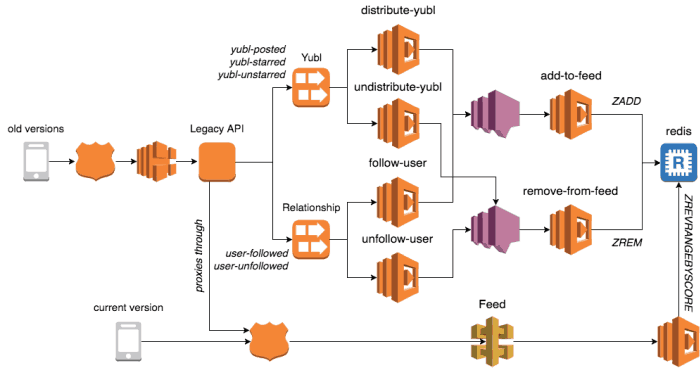
fan-out with SQS
Before the advent of AWS Lambda, this type of workload is often carried out with SQS. Unfortunately SQS is not one of the supported event sources for Lambda, which puts it in a massive disadvantage here.
That said, SQS itself is still a good choice for distributing tasks and if your subtasks take longer than 5 minutes to complete (the max execution time for Lambda) you might still have to find a way to make the SQS + Lambda setup work.
Let me explain what I mean.
First, it’s possible for a Lambda function to go beyond the 5 min execution time limit by writing it as a recursive function. However, the original invocation (triggered by SNS) has to signal whether or not the SNS message was successfully processed, but that information is only available at the end of the recursion!
With SQS, you have a message handle that can be passed along during recursion. The recursed invocation can then use the handle to:
- extend the visibility timeout for the message so another SQS poller does not receive it whilst we’re still processing the message
- delete the message if we’re able to successfully process it
A while back, I prototyped an architecture for processing SQS messages using recursive Lambda functions. The architecture allows for elastically scaling up and down the no. of pollers based on the size of the backlog (or whatever CloudWatch metric you choose to scale on).
You can read all about it here.
I don’t believe it lowers the bar of entry for the SQS + Lambda setup enough for regular use, not to mention the additional cost of running a Lambda function 24/7 for polling SQS.
The good news is that, AWS announced that SQS event source is coming to Lambda! So hopefully in the future you won’t need workarounds like the one I created to use Lambda with SQS.
What about Kinesis or DynamoDB Streams?
Personally I don’t feel these are great options, because the degree of parallelism is constrained by the no. of shards. Whilst you can increase the no. of shards, it’s a really expensive way to get extra parallelism, especially given the way resharding works in Kinesis Streams – after splitting an existing shard, the old shard is still around for at least 24 hours (based on your retention policy) and you’ll continue to pay for it.
Therefore, dynamically adjusting the no. of shards to scale up and down the degree of parallelism you’re after can incur lots unnecessary cost.
With DynamoDB Streams, you don’t even have the option to reshard the stream – it’s a managed stream that reshards as it sees fit.
fan-in: collecting results from workers
When the ventilator
function partition the original task into many subtasks, it can also include two identifiers with each subtask?—?one for the top level job, and one for the subtask. When the subtasks are completed, you can use the identifiers to record their results against.
For example, you might use a DynamoDB table to store these results. But bare in mind that DynamoDB has a max item size of 400KB including attribute names.
Alternatively, you may also consider storing the results in S3, which has a max object size of a whopping 5TB! For example, you can store the results as the following:
bucket/job_id/task_01.json bucket/job_id/task_02.json bucket/job_id/task_03.json ...
Note that in both cases we’re prone to experience hot partitions – large no. of writes against the same DynamoDB hash key or S3 prefix.
To mitigate this negative effect, be sure to use a GUID for the job ID.
Depending on the volume of write operations you need to perform against S3, you might need to tweak the approach. For example:
- partition the bucket with top level folders and place results in to the correct folder based on hash value of the job ID
bucket/01/job_id_001/task_01.json bucket/01/job_id_001/task_02.json bucket/01/job_id_001/task_03.json ...
- store the results in easily hashable but unstructured way in S3, but also record references to them in DynamoDB table
bucket/ffa7046a-105e-4a00-82e6-849cd36c303b.json bucket/8fb59303-d379-44b0-8df6-7a479d58e387.json bucket/ba6d48b6-bf63-46d1-8c15-10066a1ceaee.json ...
fan-in: tracking overall progress
When the ventilator
function runs and partitions the expensive task into lots small subtasks, it should also record the total no. of subtasks. This way, it allows each invocation of the worker
function to atomically decrement the count, until it reaches 0.
The invocation that sees the count reach 0 is then responsible for signalling that all the subtasks are complete. It can do this in many ways, perhaps by publishing a message to another SNS topic so the worker
function is decoupled from whatever post steps that need to happen to aggregate the individual results.
(wait, so are we back to the pub-sub pattern again?) maybe ;-)
At this point, the sink
function (or reducer, as it’s called in the context of a map-reduce job) would be invoked. Seeing as you’re likely to have a large no. of results to collect, it might be a good idea to also write the sink
function as a recursive function too.
Anyway, these are just a few of the ways I can think of to implement the push-poll
pattern with AWS Lambda. Let me know in the comments if I have missed any obvious alternatives.
Whenever you’re ready, here are 4 ways I can help you:
- Production-Ready Serverless: Join 20+ AWS Heroes & Community Builders and 1000+ other students in levelling up your serverless game. This is your one-stop shop for quickly levelling up your serverless skills.
- Do you want to know how to test serverless architectures with a fast dev & test loop? Check out my latest course, Testing Serverless Architectures and learn the smart way to test serverless.
- I help clients launch product ideas, improve their development processes and upskill their teams. If you’d like to work together, then let’s get in touch.
- Join my community on Discord, ask questions, and join the discussion on all things AWS and Serverless.